11. Self-calibrated CS-pMR image reconstruction from undersampled Cartesian data#
In this tutorial we will reconstruct an MRI image from Cartesian undersampled kspace data.
Let us denote \(\Omega\) the undersampling mask, the under-sampled Fourier transform now reads \(F_{\Omega}\). We use the toy datasets available in pysap, more specifically a 2D brain slice and under-sampled Cartesian acquisition over 32 channels. We compare zero-order image reconstruction with self-calibrated multi-coil Compressed sensing reconstructions (analysis vs synthesis formulation) using the FISTA algorithm for the synthesis formulation and the Condat-Vu algorithm for the analysis formulation. The multicoil data \((y_\ell)_\ell\) is collected across multiple, say \(L\), channels. The sensitivity maps \((S_\ell)_\ell\) are automically calibrated from the central portion of k-space (e.g. 5%) for all channels \(\ell=1, \ldots, L\). We remind that the synthesis formulation of the non-Cartesian CS-PMRI problem reads (minimization in the sparsifying domain):
and the image solution is given by \(\widehat{x} = \Psi^*\widehat{z}\). For an orthonormal wavelet transform, we have \(n_\Psi=n\) while for a frame we may have \(n_\Psi > n\). while the analysis formulation consists in minimizing the following cost function (min. in the image domain):
Author: Chaithya G R & Philippe Ciuciu
Date: 01/06/2021, update: 02/13/2024
Target: ATSI MSc students, Paris-Saclay University
#DISPLAY BRAIN PHANTOM
%matplotlib inline
# Package import
from mri.operators import FFT, WaveletN
from mri.operators.utils import convert_mask_to_locations
from mri.reconstructors.utils.extract_sensitivity_maps \
import get_Smaps, extract_k_space_center_and_locations
from mri.reconstructors import SelfCalibrationReconstructor
from pysap.data import get_sample_data
# Third party import
from modopt.math.metrics import ssim
from modopt.opt.linear import Identity
from modopt.opt.proximity import SparseThreshold
import numpy as np
import matplotlib.pyplot as plt
/volatile/github-ci-mind-inria/gpu_mind_runner/_work/mri-acq-recon-book/mri-acq-recon-book/venv/lib/python3.10/site-packages/tqdm/auto.py:21: TqdmWarning: IProgress not found. Please update jupyter and ipywidgets. See https://ipywidgets.readthedocs.io/en/stable/user_install.html
from .autonotebook import tqdm as notebook_tqdm
Loading input data#
# Loading input data
cartesian_ref_image = get_sample_data('2d-pmri')
image = np.linalg.norm(cartesian_ref_image, axis=0)
# Obtain MRI cartesian mask
mask = get_sample_data("cartesian-mri-mask").data
# View Input
plt.subplot(1, 2, 1)
plt.imshow(np.abs(image), cmap='gray')
plt.title("MRI Data")
plt.subplot(1, 2, 2)
plt.imshow(mask, cmap='gray')
plt.title("K-space Sampling Mask")
plt.show()
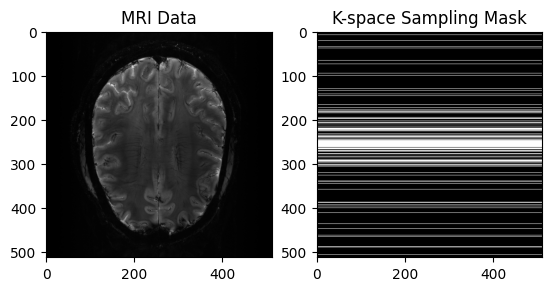
mask_sampled = np.where(mask==1)
print(512**2/np.size(mask_sampled))
2.6122448979591835
# Alternative design of 1D VDS
from mrinufft.trajectories.tools import get_random_loc_1d
phase_encoding_locs = get_random_loc_1d(image.shape[0], accel=8, center_prop=0.1, pdf='gaussian')
print(phase_encoding_locs, min(phase_encoding_locs), max(phase_encoding_locs))
phase_encoding_locs = ((phase_encoding_locs +0.5) * image.shape[0]).astype(int)
mask = np.zeros(image.shape, dtype=bool)
mask[phase_encoding_locs] = 1
# View Input
plt.subplot(1, 2, 1)
plt.imshow(np.abs(image), cmap='gray')
plt.title("MRI Data")
plt.subplot(1, 2, 2)
plt.imshow(mask, cmap='gray')
plt.title("K-space Sampling Mask")
plt.show()
[ 0. 0.00195312 -0.00195312 0.00390625 -0.00390625 0.00585938
-0.00585938 0.0078125 -0.0078125 0.00976562 -0.00976562 0.01171875
-0.01171875 0.01367188 -0.01367188 0.015625 -0.015625 0.01757812
-0.01757812 0.01953125 -0.01953125 0.02148438 -0.02148438 0.0234375
-0.0234375 0.02539062 -0.02539062 0.02734375 -0.02734375 0.02929688
-0.02929688 0.03125 -0.03125 0.03320312 -0.03320312 0.03515625
-0.03515625 0.03710938 -0.03710938 0.0390625 -0.0390625 0.04101562
-0.04101562 0.04296875 -0.04296875 0.04492188 -0.04492188 0.046875
-0.046875 0.05664062 -0.04882812 0.0625 -0.05078125 0.06445312
-0.05859375 0.07617188 -0.06445312 0.08007812 -0.06835938 0.08789062
-0.0703125 0.08984375 -0.07226562 0.09375 -0.078125 0.10742188
-0.08007812 0.13085938 -0.08203125 0.15039062 -0.0859375 0.15429688
-0.09179688 0.15625 -0.09375 0.15820312 -0.09570312 0.16601562
-0.11132812 0.18164062 -0.11914062 0.18554688 -0.12304688 0.20703125
-0.125 0.27539062 -0.1328125 -0.13476562 -0.13867188 -0.15039062
-0.15429688 -0.15625 -0.16015625 -0.1796875 -0.18164062 -0.19140625
-0.20117188 -0.2265625 -0.23046875 -0.23828125 -0.24414062 -0.25390625
-0.26953125 -0.27734375 -0.29101562 -0.33398438 -0.40039062 -0.40234375] -0.40234375 0.275390625
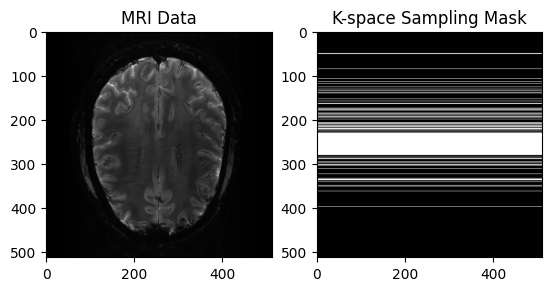
Generate the kspace#
From the 2D brain slice and the acquisition mask, we retrospectively undersample the k-space using a cartesian acquisition mask. We then reconstruct the zero order solution as a baseline
# Get the locations of the kspace samples and the associated observations
fourier_op = FFT(mask=mask, shape=image.shape,
n_coils=cartesian_ref_image.shape[0])
kspace_obs = fourier_op.op(cartesian_ref_image)
# Zero order solution
zero_soln = np.linalg.norm(fourier_op.adj_op(kspace_obs), axis=0)
base_ssim = ssim(zero_soln, image)
plt.imshow(np.abs(zero_soln), cmap='gray')
plt.title('Zero Order Solution : SSIM = ' + str(np.around(base_ssim, 3)))
plt.show()
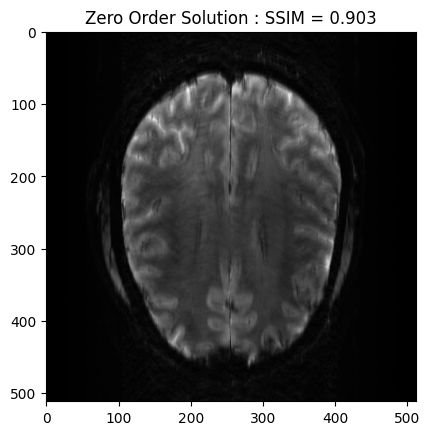
# Obtain SMaps
kspace_loc = convert_mask_to_locations(mask)
Smaps, SOS = get_Smaps(
k_space=kspace_obs,
img_shape=fourier_op.shape,
samples=kspace_loc,
thresh=(0.01, 0.01), # The cutoff threshold in each kspace direction
# between 0 and kspace_max (0.5)
min_samples=kspace_loc.min(axis=0),
max_samples=kspace_loc.max(axis=0),
mode='gridding',
method='linear',
n_cpu=-1,
)
h=3;w=5;
f, axs = plt.subplots(h,w)
for i in range(h):
for j in range(w):
axs[i, j].imshow(np.abs(Smaps[3 * i + j]))
axs[i, j].axis('off')
plt.subplots_adjust(wspace=0,hspace=0)
plt.show()
/volatile/github-ci-mind-inria/gpu_mind_runner/_work/mri-acq-recon-book/mri-acq-recon-book/venv/lib/python3.10/site-packages/mri/reconstructors/utils/extract_sensitivity_maps.py:86: UserWarning: Data Values seem to have rank 3 (>2). Using is_fft for now.
warnings.warn('Data Values seem to have rank '
[Parallel(n_jobs=-1)]: Using backend LokyBackend with 48 concurrent workers.
[Parallel(n_jobs=-1)]: Done 3 out of 32 | elapsed: 3.8s remaining: 36.9s
[Parallel(n_jobs=-1)]: Done 32 out of 32 | elapsed: 5.1s finished
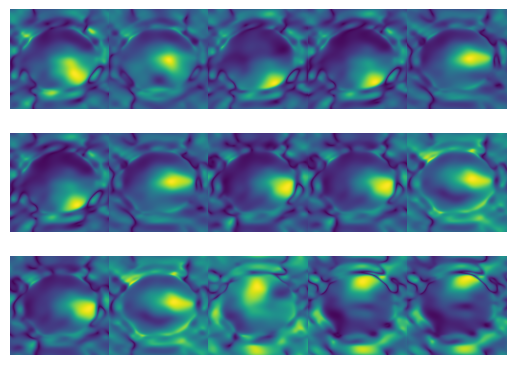
FISTA optimization#
We now want to refine the zero order solution using a FISTA optimization. The cost function is set to Proximity Cost + Gradient Cost
# Setup the operators
linear_op = WaveletN(
wavelet_name='sym8',
nb_scale=4,
)
regularizer_op = SparseThreshold(Identity(), 1.5e-8, thresh_type="soft")
# Setup Reconstructor
reconstructor = SelfCalibrationReconstructor(
fourier_op=fourier_op,
linear_op=linear_op,
regularizer_op=regularizer_op,
gradient_formulation='synthesis',
kspace_portion=0.01,
verbose=1,
)
image_rec, costs, metrics = reconstructor.reconstruct(
kspace_data=kspace_obs,
optimization_alg='fista',
num_iterations=200,
)
recon_ssim = ssim(image_rec, image)
plt.imshow(np.abs(image_rec), cmap='gray')
plt.title('Iterative FISTA Reconstruction : SSIM = ' + str(np.around(recon_ssim, 3)))
plt.show()
WARNING: Making input data immutable.
Lipschitz constant is 1.0705663895934379
WARNING: Making input data immutable.
The lipschitz constraint is satisfied
- mu: 1.5e-08
- lipschitz constant: 1.0705663895934379
- data: (512, 512)
- wavelet: <mri.operators.linear.wavelet.WaveletN object at 0x7f8823d4da50> - 4
- max iterations: 200
- image variable shape: (512, 512)
- alpha variable shape: (291721,)
----------------------------------------
Starting optimization...
0%| | 0/200 [00:00<?, ?it/s]
0%| | 1/200 [00:01<03:27, 1.04s/it]
1%| | 2/200 [00:02<03:26, 1.04s/it]
2%|▏ | 3/200 [00:03<03:20, 1.02s/it]
2%|▏ | 4/200 [00:04<03:17, 1.01s/it]
2%|▎ | 5/200 [00:05<03:16, 1.01s/it]
3%|▎ | 6/200 [00:06<03:13, 1.00it/s]
4%|▎ | 7/200 [00:07<03:12, 1.00it/s]
4%|▍ | 8/200 [00:08<03:10, 1.01it/s]
4%|▍ | 9/200 [00:09<03:09, 1.01it/s]
5%|▌ | 10/200 [00:10<03:08, 1.01it/s]
6%|▌ | 11/200 [00:10<03:07, 1.01it/s]
6%|▌ | 12/200 [00:11<03:06, 1.01it/s]
6%|▋ | 13/200 [00:12<03:05, 1.01it/s]
7%|▋ | 14/200 [00:13<03:04, 1.01it/s]
8%|▊ | 15/200 [00:14<03:03, 1.01it/s]
8%|▊ | 16/200 [00:15<03:02, 1.01it/s]
8%|▊ | 17/200 [00:16<03:01, 1.01it/s]
9%|▉ | 18/200 [00:17<03:00, 1.01it/s]
10%|▉ | 19/200 [00:18<02:59, 1.01it/s]
10%|█ | 20/200 [00:19<02:58, 1.01it/s]
10%|█ | 21/200 [00:20<02:57, 1.01it/s]
11%|█ | 22/200 [00:21<02:56, 1.01it/s]
12%|█▏ | 23/200 [00:22<02:55, 1.01it/s]
12%|█▏ | 24/200 [00:23<02:54, 1.01it/s]
12%|█▎ | 25/200 [00:24<02:53, 1.01it/s]
13%|█▎ | 26/200 [00:25<02:52, 1.01it/s]
14%|█▎ | 27/200 [00:26<02:51, 1.01it/s]
14%|█▍ | 28/200 [00:27<02:50, 1.01it/s]
14%|█▍ | 29/200 [00:28<02:49, 1.01it/s]
15%|█▌ | 30/200 [00:29<02:48, 1.01it/s]
16%|█▌ | 31/200 [00:30<02:47, 1.01it/s]
16%|█▌ | 32/200 [00:31<02:46, 1.01it/s]
16%|█▋ | 33/200 [00:32<02:45, 1.01it/s]
17%|█▋ | 34/200 [00:33<02:44, 1.01it/s]
18%|█▊ | 35/200 [00:34<02:43, 1.01it/s]
18%|█▊ | 36/200 [00:35<02:43, 1.01it/s]
18%|█▊ | 37/200 [00:36<02:41, 1.01it/s]
19%|█▉ | 38/200 [00:37<02:40, 1.01it/s]
20%|█▉ | 39/200 [00:38<02:39, 1.01it/s]
20%|██ | 40/200 [00:39<02:38, 1.01it/s]
20%|██ | 41/200 [00:40<02:37, 1.01it/s]
21%|██ | 42/200 [00:41<02:36, 1.01it/s]
22%|██▏ | 43/200 [00:42<02:35, 1.01it/s]
22%|██▏ | 44/200 [00:43<02:34, 1.01it/s]
22%|██▎ | 45/200 [00:44<02:33, 1.01it/s]
23%|██▎ | 46/200 [00:45<02:32, 1.01it/s]
24%|██▎ | 47/200 [00:46<02:31, 1.01it/s]
24%|██▍ | 48/200 [00:47<02:30, 1.01it/s]
24%|██▍ | 49/200 [00:48<02:29, 1.01it/s]
25%|██▌ | 50/200 [00:49<02:31, 1.01s/it]
26%|██▌ | 51/200 [00:50<02:34, 1.03s/it]
26%|██▌ | 52/200 [00:51<02:31, 1.02s/it]
26%|██▋ | 53/200 [00:52<02:29, 1.01s/it]
27%|██▋ | 54/200 [00:53<02:27, 1.01s/it]
28%|██▊ | 55/200 [00:54<02:25, 1.00s/it]
28%|██▊ | 56/200 [00:55<02:23, 1.00it/s]
28%|██▊ | 57/200 [00:56<02:22, 1.00it/s]
29%|██▉ | 58/200 [00:57<02:21, 1.00it/s]
30%|██▉ | 59/200 [00:58<02:20, 1.01it/s]
30%|███ | 60/200 [00:59<02:19, 1.01it/s]
30%|███ | 61/200 [01:00<02:18, 1.01it/s]
31%|███ | 62/200 [01:01<02:17, 1.01it/s]
32%|███▏ | 63/200 [01:02<02:15, 1.01it/s]
32%|███▏ | 64/200 [01:03<02:14, 1.01it/s]
32%|███▎ | 65/200 [01:04<02:13, 1.01it/s]
33%|███▎ | 66/200 [01:05<02:13, 1.01it/s]
34%|███▎ | 67/200 [01:06<02:11, 1.01it/s]
34%|███▍ | 68/200 [01:07<02:11, 1.01it/s]
34%|███▍ | 69/200 [01:08<02:10, 1.01it/s]
35%|███▌ | 70/200 [01:09<02:09, 1.01it/s]
36%|███▌ | 71/200 [01:10<02:08, 1.01it/s]
36%|███▌ | 72/200 [01:11<02:07, 1.01it/s]
36%|███▋ | 73/200 [01:12<02:06, 1.01it/s]
37%|███▋ | 74/200 [01:13<02:05, 1.01it/s]
38%|███▊ | 75/200 [01:14<02:04, 1.01it/s]
38%|███▊ | 76/200 [01:15<02:03, 1.01it/s]
38%|███▊ | 77/200 [01:16<02:02, 1.01it/s]
39%|███▉ | 78/200 [01:17<02:01, 1.00it/s]
40%|███▉ | 79/200 [01:18<02:00, 1.01it/s]
40%|████ | 80/200 [01:19<01:59, 1.00it/s]
40%|████ | 81/200 [01:20<01:58, 1.00it/s]
41%|████ | 82/200 [01:21<01:57, 1.00it/s]
42%|████▏ | 83/200 [01:22<01:57, 1.00s/it]
42%|████▏ | 84/200 [01:23<01:56, 1.00s/it]
42%|████▎ | 85/200 [01:24<01:56, 1.01s/it]
43%|████▎ | 86/200 [01:25<01:55, 1.02s/it]
44%|████▎ | 87/200 [01:26<01:55, 1.02s/it]
44%|████▍ | 88/200 [01:27<01:54, 1.03s/it]
44%|████▍ | 89/200 [01:28<01:54, 1.03s/it]
45%|████▌ | 90/200 [01:29<01:52, 1.03s/it]
46%|████▌ | 91/200 [01:30<01:51, 1.03s/it]
46%|████▌ | 92/200 [01:31<01:51, 1.03s/it]
46%|████▋ | 93/200 [01:32<01:50, 1.03s/it]
47%|████▋ | 94/200 [01:33<01:48, 1.03s/it]
48%|████▊ | 95/200 [01:34<01:48, 1.03s/it]
48%|████▊ | 96/200 [01:36<01:47, 1.04s/it]
48%|████▊ | 97/200 [01:37<01:46, 1.03s/it]
49%|████▉ | 98/200 [01:38<01:45, 1.03s/it]
50%|████▉ | 99/200 [01:39<01:44, 1.03s/it]
50%|█████ | 100/200 [01:40<01:42, 1.03s/it]
50%|█████ | 101/200 [01:41<01:42, 1.03s/it]
51%|█████ | 102/200 [01:42<01:41, 1.04s/it]
52%|█████▏ | 103/200 [01:43<01:40, 1.03s/it]
52%|█████▏ | 104/200 [01:44<01:38, 1.03s/it]
52%|█████▎ | 105/200 [01:45<01:37, 1.03s/it]
53%|█████▎ | 106/200 [01:46<01:36, 1.03s/it]
54%|█████▎ | 107/200 [01:47<01:35, 1.03s/it]
54%|█████▍ | 108/200 [01:48<01:34, 1.03s/it]
55%|█████▍ | 109/200 [01:49<01:34, 1.03s/it]
55%|█████▌ | 110/200 [01:50<01:32, 1.03s/it]
56%|█████▌ | 111/200 [01:51<01:31, 1.03s/it]
56%|█████▌ | 112/200 [01:52<01:30, 1.02s/it]
56%|█████▋ | 113/200 [01:53<01:29, 1.03s/it]
57%|█████▋ | 114/200 [01:54<01:28, 1.03s/it]
57%|█████▊ | 115/200 [01:55<01:27, 1.03s/it]
58%|█████▊ | 116/200 [01:56<01:26, 1.03s/it]
58%|█████▊ | 117/200 [01:57<01:25, 1.03s/it]
59%|█████▉ | 118/200 [01:58<01:24, 1.03s/it]
60%|█████▉ | 119/200 [01:59<01:23, 1.02s/it]
60%|██████ | 120/200 [02:00<01:22, 1.03s/it]
60%|██████ | 121/200 [02:01<01:21, 1.03s/it]
61%|██████ | 122/200 [02:02<01:20, 1.03s/it]
62%|██████▏ | 123/200 [02:03<01:19, 1.03s/it]
62%|██████▏ | 124/200 [02:04<01:18, 1.03s/it]
62%|██████▎ | 125/200 [02:05<01:16, 1.03s/it]
63%|██████▎ | 126/200 [02:06<01:15, 1.02s/it]
64%|██████▎ | 127/200 [02:07<01:14, 1.02s/it]
64%|██████▍ | 128/200 [02:08<01:13, 1.02s/it]
64%|██████▍ | 129/200 [02:09<01:12, 1.02s/it]
65%|██████▌ | 130/200 [02:10<01:12, 1.03s/it]
66%|██████▌ | 131/200 [02:11<01:11, 1.03s/it]
66%|██████▌ | 132/200 [02:13<01:09, 1.03s/it]
66%|██████▋ | 133/200 [02:14<01:08, 1.03s/it]
67%|██████▋ | 134/200 [02:15<01:07, 1.02s/it]
68%|██████▊ | 135/200 [02:16<01:06, 1.02s/it]
68%|██████▊ | 136/200 [02:17<01:05, 1.02s/it]
68%|██████▊ | 137/200 [02:18<01:04, 1.03s/it]
69%|██████▉ | 138/200 [02:19<01:03, 1.03s/it]
70%|██████▉ | 139/200 [02:20<01:02, 1.02s/it]
70%|███████ | 140/200 [02:21<01:00, 1.01s/it]
70%|███████ | 141/200 [02:22<00:59, 1.00s/it]
71%|███████ | 142/200 [02:23<00:58, 1.00s/it]
72%|███████▏ | 143/200 [02:24<00:57, 1.01s/it]
72%|███████▏ | 144/200 [02:25<00:56, 1.01s/it]
72%|███████▎ | 145/200 [02:26<00:56, 1.02s/it]
73%|███████▎ | 146/200 [02:27<00:55, 1.02s/it]
74%|███████▎ | 147/200 [02:28<00:54, 1.02s/it]
74%|███████▍ | 148/200 [02:29<00:53, 1.02s/it]
74%|███████▍ | 149/200 [02:30<00:52, 1.02s/it]
75%|███████▌ | 150/200 [02:31<00:51, 1.02s/it]
76%|███████▌ | 151/200 [02:32<00:50, 1.02s/it]
76%|███████▌ | 152/200 [02:33<00:49, 1.03s/it]
76%|███████▋ | 153/200 [02:34<00:48, 1.03s/it]
77%|███████▋ | 154/200 [02:35<00:47, 1.03s/it]
78%|███████▊ | 155/200 [02:36<00:46, 1.03s/it]
78%|███████▊ | 156/200 [02:37<00:45, 1.03s/it]
78%|███████▊ | 157/200 [02:38<00:44, 1.03s/it]
79%|███████▉ | 158/200 [02:39<00:43, 1.03s/it]
80%|███████▉ | 159/200 [02:40<00:42, 1.03s/it]
80%|████████ | 160/200 [02:41<00:41, 1.04s/it]
80%|████████ | 161/200 [02:42<00:40, 1.03s/it]
81%|████████ | 162/200 [02:43<00:39, 1.03s/it]
82%|████████▏ | 163/200 [02:44<00:38, 1.03s/it]
82%|████████▏ | 164/200 [02:45<00:37, 1.03s/it]
82%|████████▎ | 165/200 [02:46<00:35, 1.03s/it]
83%|████████▎ | 166/200 [02:47<00:35, 1.03s/it]
84%|████████▎ | 167/200 [02:48<00:34, 1.04s/it]
84%|████████▍ | 168/200 [02:49<00:33, 1.03s/it]
84%|████████▍ | 169/200 [02:50<00:31, 1.03s/it]
85%|████████▌ | 170/200 [02:51<00:30, 1.03s/it]
86%|████████▌ | 171/200 [02:53<00:29, 1.03s/it]
86%|████████▌ | 172/200 [02:54<00:28, 1.03s/it]
86%|████████▋ | 173/200 [02:55<00:27, 1.02s/it]
87%|████████▋ | 174/200 [02:56<00:26, 1.01s/it]
88%|████████▊ | 175/200 [02:57<00:25, 1.00s/it]
88%|████████▊ | 176/200 [02:58<00:24, 1.00s/it]
88%|████████▊ | 177/200 [02:58<00:23, 1.00s/it]
89%|████████▉ | 178/200 [02:59<00:21, 1.00it/s]
90%|████████▉ | 179/200 [03:00<00:20, 1.00it/s]
90%|█████████ | 180/200 [03:01<00:19, 1.00it/s]
90%|█████████ | 181/200 [03:02<00:18, 1.00it/s]
91%|█████████ | 182/200 [03:03<00:17, 1.00it/s]
92%|█████████▏| 183/200 [03:04<00:16, 1.00it/s]
92%|█████████▏| 184/200 [03:05<00:15, 1.01it/s]
92%|█████████▎| 185/200 [03:06<00:14, 1.01it/s]
93%|█████████▎| 186/200 [03:07<00:13, 1.01it/s]
94%|█████████▎| 187/200 [03:08<00:12, 1.01it/s]
94%|█████████▍| 188/200 [03:09<00:11, 1.01it/s]
94%|█████████▍| 189/200 [03:10<00:10, 1.01it/s]
95%|█████████▌| 190/200 [03:11<00:09, 1.01it/s]
96%|█████████▌| 191/200 [03:12<00:08, 1.01it/s]
96%|█████████▌| 192/200 [03:13<00:07, 1.01it/s]
96%|█████████▋| 193/200 [03:14<00:06, 1.01it/s]
97%|█████████▋| 194/200 [03:15<00:05, 1.01it/s]
98%|█████████▊| 195/200 [03:16<00:04, 1.01it/s]
98%|█████████▊| 196/200 [03:17<00:03, 1.01it/s]
98%|█████████▊| 197/200 [03:18<00:02, 1.01it/s]
99%|█████████▉| 198/200 [03:19<00:01, 1.01it/s]
100%|█████████▉| 199/200 [03:20<00:00, 1.01it/s]
100%|██████████| 200/200 [03:21<00:00, 1.01it/s]
100%|██████████| 200/200 [03:21<00:00, 1.01s/it]
- final iteration number: 200
- final log10 cost value: 6.0
- converged: False
Done.
Execution time: 201.84669504594058 seconds
----------------------------------------
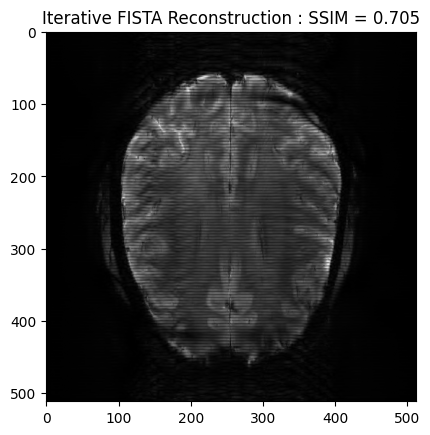
POGM reconstruction#
image_rec2, costs, metrics = reconstructor.reconstruct(
kspace_data=kspace_obs,
optimization_alg='fista',
num_iterations=200,
)
recon2_ssim = ssim(image_rec2, image)
plt.imshow(np.abs(image_rec2), cmap='gray')
plt.title('Iterative POGM Reconstruction : SSIM = ' + str(np.around(recon2_ssim, 3)))
plt.show()
WARNING: Making input data immutable.
Lipschitz constant is 1.0703795956517566
The lipschitz constraint is satisfied
- mu: 1.5e-08
- lipschitz constant: 1.0703795956517566
- data: (512, 512)
- wavelet: <mri.operators.linear.wavelet.WaveletN object at 0x7f8823d4da50> - 4
- max iterations: 200
- image variable shape: (512, 512)
- alpha variable shape: (291721,)
----------------------------------------
Starting optimization...
0%| | 0/200 [00:00<?, ?it/s]
0%| | 1/200 [00:01<03:21, 1.01s/it]
1%| | 2/200 [00:02<03:18, 1.00s/it]
2%|▏ | 3/200 [00:02<03:16, 1.00it/s]
2%|▏ | 4/200 [00:03<03:15, 1.00it/s]
2%|▎ | 5/200 [00:04<03:14, 1.00it/s]
3%|▎ | 6/200 [00:05<03:13, 1.00it/s]
4%|▎ | 7/200 [00:06<03:12, 1.01it/s]
4%|▍ | 8/200 [00:07<03:10, 1.01it/s]
4%|▍ | 9/200 [00:08<03:10, 1.00it/s]
5%|▌ | 10/200 [00:09<03:09, 1.00it/s]
6%|▌ | 11/200 [00:10<03:08, 1.00it/s]
6%|▌ | 12/200 [00:11<03:07, 1.00it/s]
6%|▋ | 13/200 [00:12<03:05, 1.01it/s]
7%|▋ | 14/200 [00:13<03:04, 1.01it/s]
8%|▊ | 15/200 [00:14<03:04, 1.00it/s]
8%|▊ | 16/200 [00:15<03:03, 1.00it/s]
8%|▊ | 17/200 [00:16<03:01, 1.01it/s]
9%|▉ | 18/200 [00:17<03:01, 1.01it/s]
10%|▉ | 19/200 [00:18<02:59, 1.01it/s]
10%|█ | 20/200 [00:19<02:59, 1.00it/s]
10%|█ | 21/200 [00:20<02:58, 1.00it/s]
11%|█ | 22/200 [00:21<02:57, 1.00it/s]
12%|█▏ | 23/200 [00:22<02:56, 1.00it/s]
12%|█▏ | 24/200 [00:23<02:55, 1.00it/s]
12%|█▎ | 25/200 [00:24<02:54, 1.00it/s]
13%|█▎ | 26/200 [00:25<02:52, 1.01it/s]
14%|█▎ | 27/200 [00:26<02:51, 1.01it/s]
14%|█▍ | 28/200 [00:27<02:50, 1.01it/s]
14%|█▍ | 29/200 [00:28<02:50, 1.00it/s]
15%|█▌ | 30/200 [00:29<02:49, 1.00it/s]
16%|█▌ | 31/200 [00:30<02:48, 1.00it/s]
16%|█▌ | 32/200 [00:31<02:47, 1.00it/s]
16%|█▋ | 33/200 [00:32<02:47, 1.00s/it]
17%|█▋ | 34/200 [00:33<02:46, 1.00s/it]
18%|█▊ | 35/200 [00:34<02:45, 1.00s/it]
18%|█▊ | 36/200 [00:35<02:45, 1.01s/it]
18%|█▊ | 37/200 [00:36<02:43, 1.01s/it]
19%|█▉ | 38/200 [00:37<02:42, 1.00s/it]
20%|█▉ | 39/200 [00:38<02:41, 1.00s/it]
20%|██ | 40/200 [00:39<02:41, 1.01s/it]
20%|██ | 41/200 [00:40<02:39, 1.00s/it]
21%|██ | 42/200 [00:41<02:39, 1.01s/it]
22%|██▏ | 43/200 [00:42<02:38, 1.01s/it]
22%|██▏ | 44/200 [00:43<02:37, 1.01s/it]
22%|██▎ | 45/200 [00:44<02:36, 1.01s/it]
23%|██▎ | 46/200 [00:45<02:35, 1.01s/it]
24%|██▎ | 47/200 [00:46<02:34, 1.01s/it]
24%|██▍ | 48/200 [00:48<02:33, 1.01s/it]
24%|██▍ | 49/200 [00:49<02:34, 1.02s/it]
25%|██▌ | 50/200 [00:50<02:32, 1.02s/it]
26%|██▌ | 51/200 [00:51<02:30, 1.01s/it]
26%|██▌ | 52/200 [00:52<02:28, 1.01s/it]
26%|██▋ | 53/200 [00:53<02:27, 1.00s/it]
27%|██▋ | 54/200 [00:54<02:26, 1.00s/it]
28%|██▊ | 55/200 [00:55<02:25, 1.00s/it]
28%|██▊ | 56/200 [00:56<02:24, 1.00s/it]
28%|██▊ | 57/200 [00:57<02:23, 1.00s/it]
29%|██▉ | 58/200 [00:58<02:21, 1.00it/s]
30%|██▉ | 59/200 [00:59<02:20, 1.00it/s]
30%|███ | 60/200 [01:00<02:19, 1.00it/s]
30%|███ | 61/200 [01:01<02:18, 1.00it/s]
31%|███ | 62/200 [01:02<02:17, 1.00it/s]
32%|███▏ | 63/200 [01:03<02:17, 1.00s/it]
32%|███▏ | 64/200 [01:04<02:16, 1.00s/it]
32%|███▎ | 65/200 [01:05<02:15, 1.00s/it]
33%|███▎ | 66/200 [01:06<02:13, 1.00it/s]
34%|███▎ | 67/200 [01:07<02:12, 1.00it/s]
34%|███▍ | 68/200 [01:08<02:11, 1.00it/s]
34%|███▍ | 69/200 [01:09<02:10, 1.00it/s]
35%|███▌ | 70/200 [01:10<02:09, 1.00it/s]
36%|███▌ | 71/200 [01:11<02:08, 1.00it/s]
36%|███▌ | 72/200 [01:12<02:07, 1.00it/s]
36%|███▋ | 73/200 [01:13<02:06, 1.00it/s]
37%|███▋ | 74/200 [01:14<02:05, 1.00it/s]
38%|███▊ | 75/200 [01:15<02:04, 1.00it/s]
38%|███▊ | 76/200 [01:16<02:03, 1.00it/s]
38%|███▊ | 77/200 [01:17<02:02, 1.00it/s]
39%|███▉ | 78/200 [01:18<02:01, 1.00it/s]
40%|███▉ | 79/200 [01:18<02:00, 1.00it/s]
40%|████ | 80/200 [01:19<01:59, 1.00it/s]
40%|████ | 81/200 [01:20<01:58, 1.00it/s]
41%|████ | 82/200 [01:21<01:57, 1.00it/s]
42%|████▏ | 83/200 [01:22<01:56, 1.00it/s]
42%|████▏ | 84/200 [01:23<01:55, 1.00it/s]
42%|████▎ | 85/200 [01:24<01:54, 1.00it/s]
43%|████▎ | 86/200 [01:25<01:53, 1.01it/s]
44%|████▎ | 87/200 [01:26<01:52, 1.01it/s]
44%|████▍ | 88/200 [01:27<01:51, 1.01it/s]
44%|████▍ | 89/200 [01:28<01:50, 1.01it/s]
45%|████▌ | 90/200 [01:29<01:49, 1.00it/s]
46%|████▌ | 91/200 [01:30<01:48, 1.00it/s]
46%|████▌ | 92/200 [01:31<01:47, 1.00it/s]
46%|████▋ | 93/200 [01:32<01:46, 1.00it/s]
47%|████▋ | 94/200 [01:33<01:45, 1.01it/s]
48%|████▊ | 95/200 [01:34<01:44, 1.01it/s]
48%|████▊ | 96/200 [01:35<01:43, 1.01it/s]
48%|████▊ | 97/200 [01:36<01:41, 1.01it/s]
49%|████▉ | 98/200 [01:37<01:41, 1.01it/s]
50%|████▉ | 99/200 [01:38<01:40, 1.01it/s]
50%|█████ | 100/200 [01:39<01:39, 1.00it/s]
50%|█████ | 101/200 [01:40<01:38, 1.01it/s]
51%|█████ | 102/200 [01:41<01:37, 1.00it/s]
52%|█████▏ | 103/200 [01:42<01:36, 1.00it/s]
52%|█████▏ | 104/200 [01:43<01:35, 1.00it/s]
52%|█████▎ | 105/200 [01:44<01:35, 1.00s/it]
53%|█████▎ | 106/200 [01:45<01:33, 1.00it/s]
54%|█████▎ | 107/200 [01:46<01:32, 1.00it/s]
54%|█████▍ | 108/200 [01:47<01:31, 1.00it/s]
55%|█████▍ | 109/200 [01:48<01:30, 1.00it/s]
55%|█████▌ | 110/200 [01:49<01:29, 1.00it/s]
56%|█████▌ | 111/200 [01:50<01:28, 1.01it/s]
56%|█████▌ | 112/200 [01:51<01:27, 1.01it/s]
56%|█████▋ | 113/200 [01:52<01:26, 1.00it/s]
57%|█████▋ | 114/200 [01:53<01:25, 1.00it/s]
57%|█████▊ | 115/200 [01:54<01:24, 1.00it/s]
58%|█████▊ | 116/200 [01:55<01:23, 1.00it/s]
58%|█████▊ | 117/200 [01:56<01:22, 1.00it/s]
59%|█████▉ | 118/200 [01:57<01:21, 1.00it/s]
60%|█████▉ | 119/200 [01:58<01:20, 1.00it/s]
60%|██████ | 120/200 [01:59<01:19, 1.01it/s]
60%|██████ | 121/200 [02:00<01:18, 1.01it/s]
61%|██████ | 122/200 [02:01<01:17, 1.01it/s]
62%|██████▏ | 123/200 [02:02<01:16, 1.01it/s]
62%|██████▏ | 124/200 [02:03<01:15, 1.01it/s]
62%|██████▎ | 125/200 [02:04<01:14, 1.01it/s]
63%|██████▎ | 126/200 [02:05<01:13, 1.01it/s]
64%|██████▎ | 127/200 [02:06<01:12, 1.01it/s]
64%|██████▍ | 128/200 [02:07<01:11, 1.01it/s]
64%|██████▍ | 129/200 [02:08<01:10, 1.01it/s]
65%|██████▌ | 130/200 [02:09<01:09, 1.01it/s]
66%|██████▌ | 131/200 [02:10<01:07, 1.01it/s]
66%|██████▌ | 132/200 [02:11<01:07, 1.01it/s]
66%|██████▋ | 133/200 [02:12<01:06, 1.01it/s]
67%|██████▋ | 134/200 [02:13<01:05, 1.01it/s]
68%|██████▊ | 135/200 [02:14<01:04, 1.01it/s]
68%|██████▊ | 136/200 [02:15<01:03, 1.01it/s]
68%|██████▊ | 137/200 [02:16<01:02, 1.01it/s]
69%|██████▉ | 138/200 [02:17<01:01, 1.01it/s]
70%|██████▉ | 139/200 [02:18<01:00, 1.01it/s]
70%|███████ | 140/200 [02:19<00:59, 1.01it/s]
70%|███████ | 141/200 [02:20<00:58, 1.01it/s]
71%|███████ | 142/200 [02:21<00:57, 1.01it/s]
72%|███████▏ | 143/200 [02:22<00:56, 1.01it/s]
72%|███████▏ | 144/200 [02:23<00:55, 1.01it/s]
72%|███████▎ | 145/200 [02:24<00:54, 1.01it/s]
73%|███████▎ | 146/200 [02:25<00:53, 1.01it/s]
74%|███████▎ | 147/200 [02:26<00:52, 1.01it/s]
74%|███████▍ | 148/200 [02:27<00:51, 1.01it/s]
74%|███████▍ | 149/200 [02:28<00:50, 1.01it/s]
75%|███████▌ | 150/200 [02:29<00:49, 1.01it/s]
76%|███████▌ | 151/200 [02:30<00:48, 1.01it/s]
76%|███████▌ | 152/200 [02:31<00:47, 1.01it/s]
76%|███████▋ | 153/200 [02:32<00:46, 1.01it/s]
77%|███████▋ | 154/200 [02:33<00:45, 1.01it/s]
78%|███████▊ | 155/200 [02:34<00:44, 1.01it/s]
78%|███████▊ | 156/200 [02:35<00:43, 1.01it/s]
78%|███████▊ | 157/200 [02:36<00:42, 1.01it/s]
79%|███████▉ | 158/200 [02:37<00:41, 1.01it/s]
80%|███████▉ | 159/200 [02:38<00:40, 1.01it/s]
80%|████████ | 160/200 [02:39<00:39, 1.01it/s]
80%|████████ | 161/200 [02:40<00:38, 1.01it/s]
81%|████████ | 162/200 [02:41<00:37, 1.01it/s]
82%|████████▏ | 163/200 [02:42<00:36, 1.01it/s]
82%|████████▏ | 164/200 [02:43<00:35, 1.01it/s]
82%|████████▎ | 165/200 [02:44<00:34, 1.01it/s]
83%|████████▎ | 166/200 [02:45<00:33, 1.01it/s]
84%|████████▎ | 167/200 [02:46<00:32, 1.01it/s]
84%|████████▍ | 168/200 [02:47<00:31, 1.01it/s]
84%|████████▍ | 169/200 [02:48<00:30, 1.01it/s]
85%|████████▌ | 170/200 [02:49<00:29, 1.01it/s]
86%|████████▌ | 171/200 [02:50<00:28, 1.01it/s]
86%|████████▌ | 172/200 [02:51<00:27, 1.01it/s]
86%|████████▋ | 173/200 [02:52<00:26, 1.01it/s]
87%|████████▋ | 174/200 [02:53<00:25, 1.01it/s]
88%|████████▊ | 175/200 [02:54<00:24, 1.01it/s]
88%|████████▊ | 176/200 [02:55<00:23, 1.01it/s]
88%|████████▊ | 177/200 [02:56<00:22, 1.01it/s]
89%|████████▉ | 178/200 [02:57<00:21, 1.01it/s]
90%|████████▉ | 179/200 [02:58<00:20, 1.01it/s]
90%|█████████ | 180/200 [02:59<00:19, 1.01it/s]
90%|█████████ | 181/200 [03:00<00:18, 1.01it/s]
91%|█████████ | 182/200 [03:01<00:17, 1.01it/s]
92%|█████████▏| 183/200 [03:02<00:16, 1.01it/s]
92%|█████████▏| 184/200 [03:02<00:15, 1.01it/s]
92%|█████████▎| 185/200 [03:03<00:14, 1.01it/s]
93%|█████████▎| 186/200 [03:04<00:13, 1.01it/s]
94%|█████████▎| 187/200 [03:05<00:12, 1.01it/s]
94%|█████████▍| 188/200 [03:06<00:11, 1.01it/s]
94%|█████████▍| 189/200 [03:07<00:10, 1.01it/s]
95%|█████████▌| 190/200 [03:08<00:09, 1.01it/s]
96%|█████████▌| 191/200 [03:09<00:08, 1.01it/s]
96%|█████████▌| 192/200 [03:10<00:07, 1.01it/s]
96%|█████████▋| 193/200 [03:11<00:06, 1.01it/s]
97%|█████████▋| 194/200 [03:12<00:05, 1.01it/s]
98%|█████████▊| 195/200 [03:13<00:04, 1.01it/s]
98%|█████████▊| 196/200 [03:14<00:03, 1.01it/s]
98%|█████████▊| 197/200 [03:15<00:02, 1.01it/s]
99%|█████████▉| 198/200 [03:16<00:01, 1.01it/s]
100%|█████████▉| 199/200 [03:17<00:00, 1.01it/s]
100%|██████████| 200/200 [03:18<00:00, 1.01it/s]
100%|██████████| 200/200 [03:18<00:00, 1.01it/s]
- final iteration number: 200
- final log10 cost value: 6.0
- converged: False
Done.
Execution time: 198.77881930209696 seconds
----------------------------------------
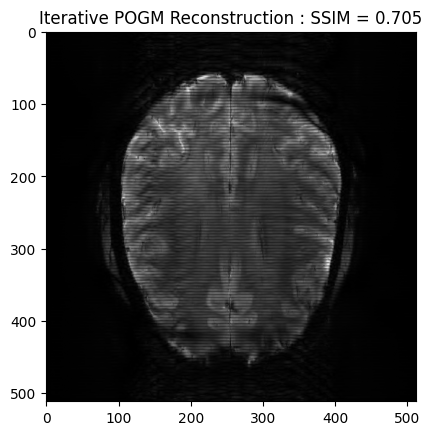